
- #Galvanic skin response graph example excel manual
- #Galvanic skin response graph example excel skin
- #Galvanic skin response graph example excel windows
Usually, higher number of peaks and greater peak values indicate higher arousal/excitement.
#Galvanic skin response graph example excel skin
Galvanic skin responses (GSR) are shown as black lines and measured in microSiemens. heart-rate variability (HRV) values of low-frequency (LF), high frequency (HF) and LF/HF ratios - higher ratio, higher mental effort.
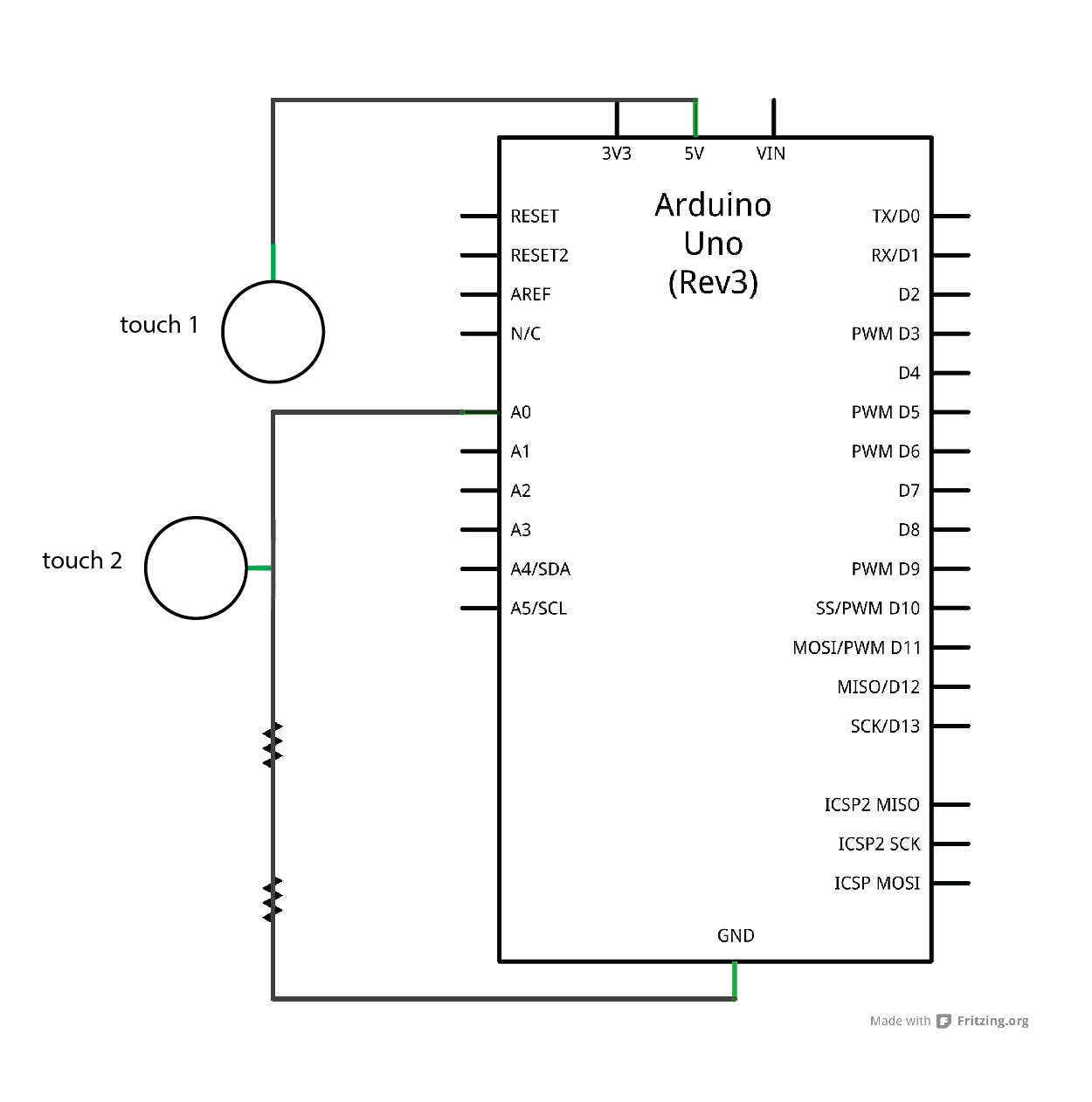
At the various confidence score peaks, we annotated: Orange line represents surprise, green line represents happiness and blue line represents sadness. In both figures, the other coloured lines represent the confidence scores for emotions. Figure 1 shows the resulting data in Excel and Figure 2 shows an example of the emotions over time plots for three cognitive tasks. This made it easier to sync the timestamps of the emotion confidence scores with video and sensors data for further analysis. Timestamps were calculated via the formula: Timestamp = Seconds/86400 and formatting the values to ‘mm:ss’ custom format. This was converted into Seconds by dividing Ticks over the Timescale (30000 Ticks): Seconds = Ticks/30000. A Tick was a measurement of time defined by the API system. By checking the files and the API documentation (now deprecated), I found that the Emotion service analysed emotion from video frames at an interval of 15000 Ticks. json files were formatted to tables in Microsoft Excel. It was modified to log the resulting confidence scores for each emotion over time from the two case study video recordings into.
#Galvanic skin response graph example excel windows
I built the application from the Windows SDK for the Emotion API using Visual Studio 2015 (C#).
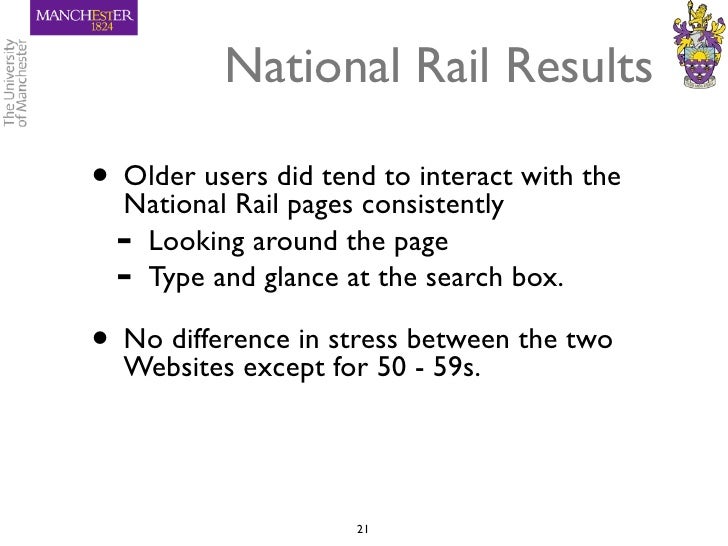
It could analyse emotions from photos and videos to give confidence scores (from 0 to 1) for the presence of eight emotions: Anger, Contempt, Disgust, Fear, Happiness, Neutral, Sadness and Surprise. Analysing Emotion from Videoįrom my review of existing tools for attaining emotion estimation from video, I selected Microsoft Emotion API (now part of Microsoft Face API) for its accuracy and ease-of-use. Thus, we aimed to explore the possibility of deriving these emotions computationally and integrating it with the rest of the data from the physiological sensors.
#Galvanic skin response graph example excel manual
However, such manual methods would be difficult and time-consuming to apply for a larger group of children, such as in a classroom. These recordings were manually coded by two researchers for behaviours and facial expressions (emotions). Video recordings were taken while the children were performing cognitive tasks of increasing mental effort to provide us with the observational data. We wanted to explain the framework through a combination of observational, physiological and performance data from two case studies (two children).

Process Behavioural and Emotional Data Analysis Background
